Financial Engineering vs. Data Science: Which Field is Right for You?
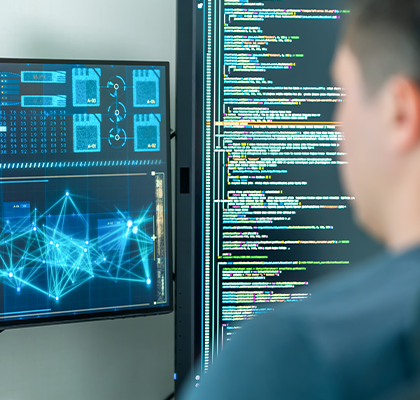
Quantitative finance combines mathematics, programming, and financial expertise to solve complex problems, using models and analytics to innovate financial products and enhance decision-making, risk management, and strategic planning. Financial Engineering and Data Science each offer unique contributions to quantitative finance. Financial Engineering focuses on creating and managing financial instruments and strategies, while Data Science utilizes large datasets and advanced analytics to extract market insights and predict trends. This article aims to clarify the differences between these two fields, helping you decide which path aligns best with your career aspirations in quantitative finance.
Key takeaways
- Overlap in Skills: Financial engineering and data science intersect in areas such as quantitative modeling, risk management, algorithmic trading, financial forecasting, product development, and big data analytics, leveraging their combined strengths to tackle complex financial challenges and drive innovation.
- Career Path Considerations: Choosing between financial engineering and data science depends on one's interests and skills. Financial engineering is suited for those passionate about financial markets and derivatives, requiring strong mathematical and analytical skills. Data science appeals to those interested in data analysis and machine learning, with a focus on large datasets and predictive insights.
- Starting a Career in Quantitative Finance: A strong foundation in mathematical modeling, programming, and financial theory is essential for careers in both fields. The Certificate in Quantitative Finance (CQF) offers practitioner-led training in these areas, positioning individuals for success in the dynamic field of quantitative finance.
What is Financial Engineering in Quant Finance?
Financial Engineering in quantitative finance is the interdisciplinary application of mathematical models, computational algorithms, and financial theory to create, analyze, and manage innovative financial products and strategies. It involves tasks such as derivative pricing, risk management, and investment portfolio optimization, aiming to solve complex financial problems and improve market efficiency. By leveraging advanced quantitative techniques, financial engineers develop solutions that address the dynamic needs of the financial industry, enhancing both strategic decision-making and risk assessment.
What is Data Science in Quant Finance?
Data Science in quantitative finance entails the application of statistical techniques, machine learning algorithms, and big data analytics to derive actionable insights from complex financial datasets. It plays a crucial role in predicting market trends, optimizing trading strategies, and enhancing decision-making processes. By utilizing advanced computational tools and data-driven models, data scientists in finance can improve risk assessment, uncover investment opportunities, and drive innovation in financial services. This approach allows for a deeper understanding of market dynamics and supports the development of more effective and efficient financial solutions.
Comparing Financial Engineering and Data Science in Quant Finance
Both Financial Engineering and Data Science play pivotal roles in quantitative finance, each with distinct focus areas, skill sets, and applications, yet both are integral to advancing financial innovation and efficiency.
Financial Engineering | Data Science | |
Core Focus Areas | Financial Engineering primarily focuses on the creation and management of financial instruments and strategies. It emphasizes derivative pricing, portfolio optimization, and risk management, leveraging mathematical models and financial theory to address complex financial problems. | Data Science concentrates on extracting insights from large datasets and predicting market trends. It uses statistical analysis and machine learning to identify patterns, optimize trading strategies, and enhance decision-making processes. |
Required Skills | Requires strong proficiency in mathematics, particularly in calculus, probability, and differential equations. Skills in computational programming (e.g., Python, C++) and a deep understanding of financial markets and instruments are essential. | Necessitates expertise in statistics, data analysis, and machine learning. Proficiency in programming languages such as Python and R, as well as experience with data manipulation tools and techniques, are critical. Understanding of financial markets is beneficial but not as central as in financial engineering. |
Applications in Quant Finance | Used in designing complex derivatives, optimizing investment portfolios, and developing risk management strategies. It plays a key role in structuring products like options, futures, and other derivative securities. | Applied in algorithmic trading, sentiment analysis, fraud detection, and credit scoring. It helps in predicting asset price movements, analyzing market behavior, and automating decision-making processes based on data-driven insights. |
Financial Engineering Careers
Financial engineering skills are essential in sectors that require sophisticated financial analysis, modeling, and product development. These include investment banks, hedge funds, asset management firms, insurance companies, and financial technology (fintech) companies. Additionally, regulatory bodies and consulting firms also seek financial engineers to navigate complex financial regulations and provide strategic advice.
Financial engineering skills are exceptionally useful for roles such as Quantitative Analyst, Risk Manager, Derivatives Trader, Portfolio Manager, Product Developer, or Financial Consultant. These roles require strong skills in statistics, programming, and machine learning, along with a solid understanding of financial markets to effectively leverage data in financial contexts.
Data Science Careers in Finance
Data science skills are highly sought after in various financial sectors, including investment banks, hedge funds, asset management firms, insurance companies, and fintech enterprises. These organizations rely on data-driven insights and predictive analytics to enhance decision-making, optimize trading strategies, and manage risks.
Data science skills are useful for roles such as Data Analyst, Data Scientist, Quantitative Researcher, Machine Learning Engineer, Algorithmic Trader, Risk Analyst, or Business Intelligence Analyst. These roles demand strong skills in statistics, programming (e.g., Python, R), and machine learning, alongside a deep understanding of financial markets and products. Data scientists in finance harness data to drive innovation, improve operational efficiency, and gain a competitive edge in the marketplace.
Where Financial Engineering and Data Science Overlap
Financial engineering and data science intersect in several key areas within the financial industry, leveraging their combined strengths to address complex challenges and drive innovation. Here are some areas where these skills overlap:
- Quantitative Modeling: Both financial engineers and data scientists use advanced quantitative models to analyze financial markets and instruments. They apply mathematical and statistical techniques to develop predictive models for pricing derivatives, optimizing portfolios, and assessing financial risk.
- Risk Management: In risk management, both disciplines contribute to identifying, quantifying, and mitigating financial risks. They develop algorithms and models to predict potential losses, assess credit risk, and ensure compliance with regulatory requirements.
- Algorithmic Trading: Algorithmic trading is a prominent area of overlap, where financial engineers design trading strategies and data scientists implement machine learning models to optimize execution, predict market movements, and enhance trading performance.
- Financial Forecasting: Both fields use statistical and machine learning models to forecast market trends, economic indicators, and asset prices. These predictive insights help in strategic decision-making and investment planning.
- Product Development: In financial product development, they collaborate to design innovative financial products and solutions. Data science enhances the analysis of consumer behavior and market trends, while financial engineering focuses on the structural design and risk assessment of these products.
- Big Data Analytics: Both disciplines utilize big data analytics to process and analyze vast amounts of financial data. They extract insights from structured and unstructured data sources to inform strategic initiatives and improve decision-making processes.
By combining the strengths of financial engineering and data science, professionals in these fields can tackle complex financial challenges, drive efficiency, and foster innovation in the rapidly evolving financial landscape.
Financial Engineering or Data Science: Which Path is Best for You?
When deciding between a career in financial engineering or data science within the finance industry, consider the following factors to determine the best fit for your skills and interests:
Why Choose Financial Engineering?
If you are passionate about financial markets, derivatives, and complex financial instruments, and enjoy applying mathematical and analytical skills to solve financial challenges, financial engineering may be the right path for you. This field generally requires a strong foundation in mathematics, finance, and programming, with a focus on quantitative modeling and risk management. If you aspire to work in roles that involve developing trading strategies, pricing complex derivatives, and managing financial risk, then financial engineering could be the one for you.
Why Choose Data Science in Finance?
If you are fascinated by data, enjoy uncovering patterns and insights through statistical analysis and machine learning, and are excited about applying these skills to financial contexts, data science could be a better fit. Proficiency in programming languages (such as Python or R), machine learning, and statistical analysis is crucial, along with the ability to work with large datasets and derive actionable insights for careers in data science in finance. So, opt for data science if you aim to leverage data analytics and machine learning to drive decision-making, enhance financial forecasting, or contribute to innovative fintech solutions.
Ultimately, choosing the best path depends on aligning your personal interests, skills, and career aspirations with the unique opportunities and challenges each field offers. By understanding these elements, you can make an informed decision that sets the foundation for a fulfilling and successful career in finance.
Getting started in Quantitative Finance
Embarking on a career in financial engineering or data science requires a strong foundation in mathematical modeling, programming, and financial theory. One effective way to gain these skills and enhance your credentials is through the Certificate in Quantitative Finance (CQF). The CQF is the world’s leading professional qualification in quant finance and machine learning. Delivered online and part-time, the practical program is designed for individuals aiming to advance their careers in quantitative finance. It provides practitioner-led training in financial mathematics, programming, and quantitative analysis, with a curriculum that is tailored to reflect the needs of the financial markets today. By pursuing the CQF, you can acquire the essential skills and knowledge needed to excel in quantitative finance, positioning yourself for a rewarding career in this dynamic and challenging field.
Download a brochure today to learn more about the CQF and how it could help you go further in your career.