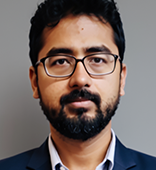
I work as a data scientist within JP Morgan’s Corporate and Investment Bank (CIB) division, building machine learning models for operational risk management. On these projects, I collaborate with clients from legal and management teams to solve business problems in their operations.
I originally come from a research background. I graduated with a PhD in physics from the University of Arizona in 2018. There, I worked as a computational physicist, studying quantum mechanical processes that take place within organic solar cells to see how we could increase their efficiency. Over time, my career aspirations changed, and I took a postdoctoral fellowship in AI and machine learning, where I began working on a project that was funded by DARPA, with researchers from the University of Arizona and University of Florida. We were evaluating how we could use AI to automate sensitivity analysis within crop models and study of physiological processes within crops. After that research experience, I decided to move into an industry where I could use my mathematical modeling and computer science expertise to study real world problems. Once I decided to make this shift into finance, I wanted to be as close to the banking business as possible, learning about the different financial instruments that people deal with, understanding what kinds of derivatives products they are interested in, and what the main challenges are within the industry.
After taking the job at JP Morgan, I enrolled on the CQF to dig into essential areas such as quantitative trading practices and mathematical models used to price assets. The skills I gained on the program have helped me to build many innovative tools to perform operational risk management tasks. As an example, one of the tools that I have developed deals with understanding the impact of regulations that are put in place by agencies across the world and seeing how regulatory processes will affect the bank’s businesses. We are then able to evaluate what the businesses need to do in terms of risk assessment and controls to comply with these regulations. This work entails machine learning models based on traditional techniques, within natural language processing theory, as well as more advanced methodologies that rely on attention-based models.
When I think back on my career, I think that making the transition from academia to industry was a massive shift. In academia, your world is often limited to interacting with people who work in research facilities in a small corner of a building located on campus. In industry, you have greater exposure to a wide range of businesses and management situations. To be effective in an environment where there are diverse goals and cultures, you must understand the problems you're trying to solve and ensure that your effort has tangible impacts. My work as a researcher was interesting but very theoretical, so it might also end up gathering dust in a library until another researcher picks it up. In a bank, typically within a month or so of working on a project, you can quantify the benefits of your actions; it is quickly measurable and has real world value.
This ties into another thing that I discovered, particularly within the context of the CQF. In quant finance, we build a lot of theoretical knowledge on how to price options, how to price bonds, and so on. However, the implementation of those concepts in the market becomes the challenge. Do they work in the real world and what one must do to take the given assumptions and other factors into account?
The CQF introduced me to the wide world of financial products, and it helped me understand them from both quantitative and practical points of view.
The CQF introduced me to the wide world of financial products, and it helped me understand them from both quantitative and practical points of view. These days, I am in discussions with several groups at the bank that are looking for AI and machine learning knowledge in combination with experience in building finance applications. From what I've seen, there is currently an emphasis on data science and statistical modeling skills, which obviously is a slight departure from the traditional quant finance world. If you don’t have a background in either mathematical concepts or machine learning as they relate to quant finance, for example, you will benefit from the CQF. As you go into the program, I would recommend being or becoming familiar with linear algebra and basic differential calculus methods. In quant finance, the skillset is quite diverse, and I don't think anyone can possess all the necessary skills at equal levels of expertise. However, the foundational domain knowledge that people expect can be learned. Having a solid background in computational methods and an advanced level of math will help an individual to perform well in interviews and on the job. It’s also great to be able to benchmark against your peers, learn from each other, and have a nice group of colleagues from your cohort and the CQF alumni network as you move forward in your professional endeavors.