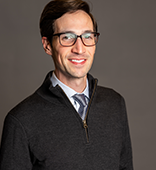
I’ve always been interested in the more quantitative and analytical side of things. I earned my undergraduate degree from a small liberal arts college, Colgate University, where I studied math and economics. After undergrad, I joined Ernst & Young’s Quantitative Advisory Services group where I specialized in derivatives pricing and risk models. After four years at EY, I transitioned to my current role at Morgan Stanley, where I focus on portfolio construction and investment research. At Morgan Stanley, I’m passionate about finding ways to incorporate machine learning and data analytics into our research process. Along the way, I earned the CFA charter and FRM designation, but neither covered quantitative topics with the depth that I was interested in. As I looked around at other educational opportunities, I was attracted to the CQF’s blend of financial engineering and machine learning content. Like many others, I also considered pursuing a full-time master’s program, but was hesitant about taking multiple years out of my career. The CQF’s flexibility seemed like a great fit for me, being online and achievable in a shorter timeframe. Just as I enrolled, the COVID pandemic shut down the US, providing me with plenty of free time to apply toward the program.
The CQF program’s flexibility was a major selling point for me. I could watch the recorded lectures early in the morning or late at night, and would rewind certain sections repeatedly until the content sunk in. Building a strong quantitative skill set has always been a priority of mine and I believe the demand for these skills will continue to grow in our industry. In my current role, I see the value to clients of a more data-driven and quantitative approach. However, since our industry can be rather slow to change, there are still many opportunities ahead for improving the way we do things.
The CQF program’s flexibility was a major selling point for me. I could watch the recorded lectures early in the morning or late at night, and would rewind certain sections repeatedly until the content sunk in.
I found that the program has a steep learning curve and felt the earlier modules to be the most difficult. As we moved into the machine learning section, however, I became intrigued by the potential applications to my role at Morgan Stanley. I decided early on that I would concentrate on ML for my final project so that I could delve deeper into the subject. My project explored the application of deep learning models to the prediction of financial asset returns. Through the project, I gained a solid understanding of the powerful machine learning libraries available in Python. Driven by my interest in the subject and helped by the ample free time given the pandemic, I endeavored to make my report as comprehensive as possible. Nevertheless, given the caliber of the other candidates, I was more than a bit surprised to learn that I earned the CQF Institute Award for the best final project in the January 2021 cohort. My advice to future CQF delegates would be to take the final project seriously. The project-based nature of the CQF means that you truly can get out of it what you put in. As far as practical learning goes, there is no substitute for sitting down and running an analysis from start to finish, with all the troubleshooting and debugging that comes with it. That's what working in quantitative research in a financial institution is all about. The CQF will definitely help you develop the right skills and approaches to put your best ideas into practice.
I earned the CQF Institute Award for the best final project in the January 2021 cohort. My advice to future CQF delegates would be to take the final project seriously. The project-based nature of the CQF means that you truly can get out of it what you put in.